AI is all the rage at the moment – but in order to get past the hype and actually implement something useful for your company, there are several things you need to take into account. We’ll look more closely at three key things in this post.
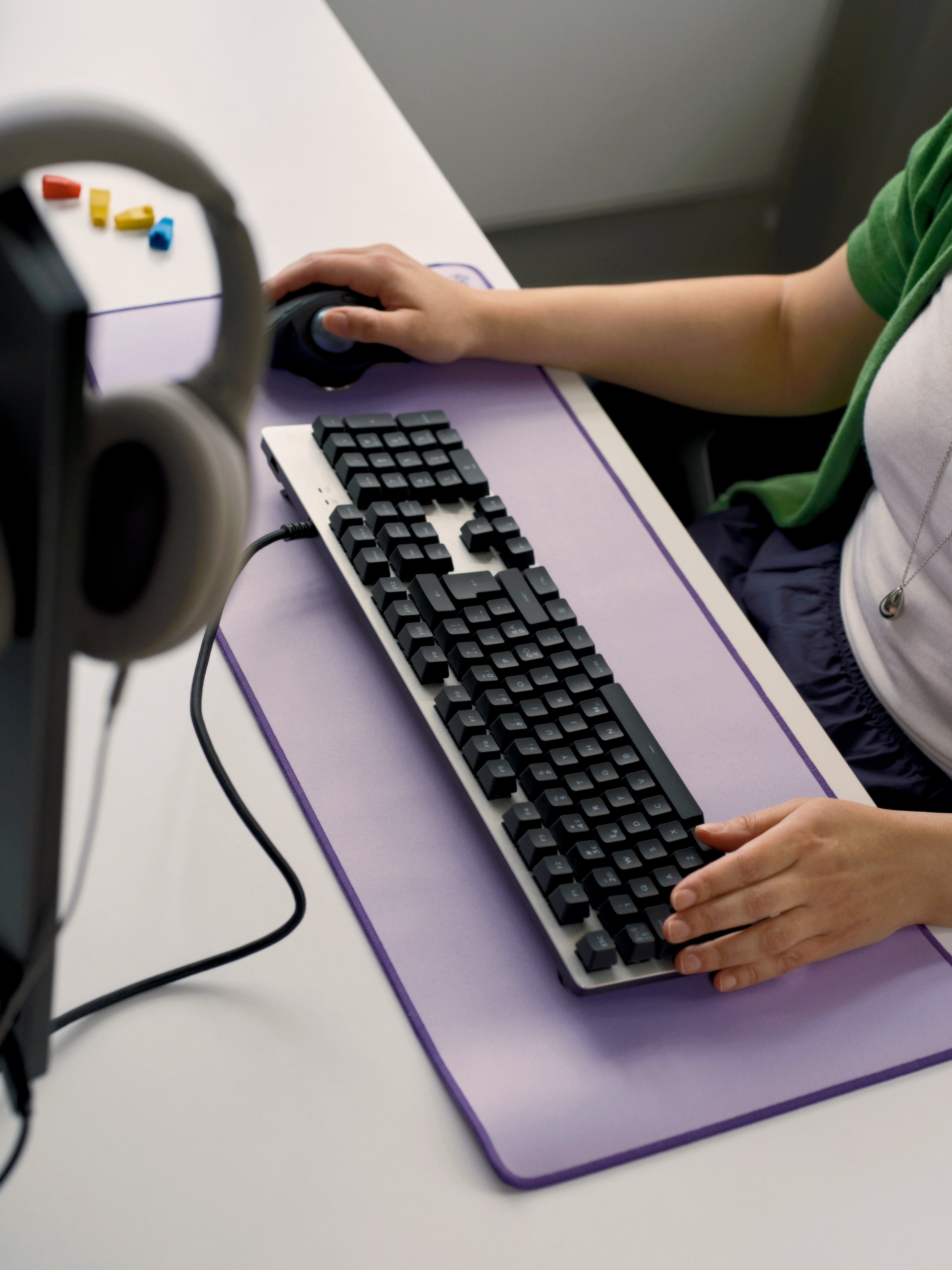
1. You need to build key capabilities
The starting point is to create an AI strategy. The strategy should set a vision for where your organization is going with AI. It should avoid specifying specific technologies – something more along the lines of “we will use AI to provide better customer service” and not “we’ll build an AI chatbot.” This gives the most flexibility to look for the right tools to move towards your vision.
From there, you need to educate people in your organization and also build the right capabilities to implement AI. This means explaining the potential of AI and then training people to use it effectively. When it comes to building capacity, outsourcing AI production can make sense or slowly building capabilities as needed.
Finally, ensuring your data is in good enough shape for AI implementation is key. This is typically the hardest thing to do as data tends to be siloed, scattered, or even in paper formats that are inaccessible to AI tools.
2. You need to implement AI in a smart way
When it comes to actually building AI tools, there are several best practices to follow. The first thing to keep in mind is to build for change. That’s because the AI field is moving fast and constantly updating. You should try and build solutions that can easily integrate new technologies.
Another key thing is to use available tools. In a field that is developing quickly, it doesn’t make sense to spend years and a huge investment developing something entirely new – chances are one of the big players in AI is going to release something similar in a short time frame.
It also makes sense to do proof of concepts first. This minimizes the initial investment and allows you to see if the basic use case for AI makes sense. In line with this, there is no need to start with major integrations to all of your data before you know if a solution works. Instead, using mocked-up data to see if the solution works in the first place.
When it comes to time to actually implement a proof of concept, gradual implementation is a smart approach. There is no need to release a fully functional product right away – for example, instead of releasing a consumer-facing chatbot to handle customer service, you could start with an AI that generates responses for your customer service agents that they can send to customers. If that works well, it can then be further refined to a point where it’s ready for customer use.
3. You need to deal with several common issues
People in organizations often feel threatened by AI and worry it will replace their jobs. It’s important to teach them how simple it is, and that there’s no magic involved. AI is simply a tool that can help employees do their job better.
AI tools are also not always ready for the task you may have in mind. For example, generative AI is prone to hallucinations (making things up), so these limitations have to be kept in mind for your use cases.
Finally, there will be new tools always coming to the market. That doesn’t mean you should wait forever – instead, it goes back to our earlier point about building for change. By making smart decisions at the start of a project, you can design modular solutions that will allow the integration of new technologies as they arrive.
💡Read our Generative AI guide to learn more!
Are you interested in finding out how AI can be put to use in your organization? Contact us to discuss more. 👇
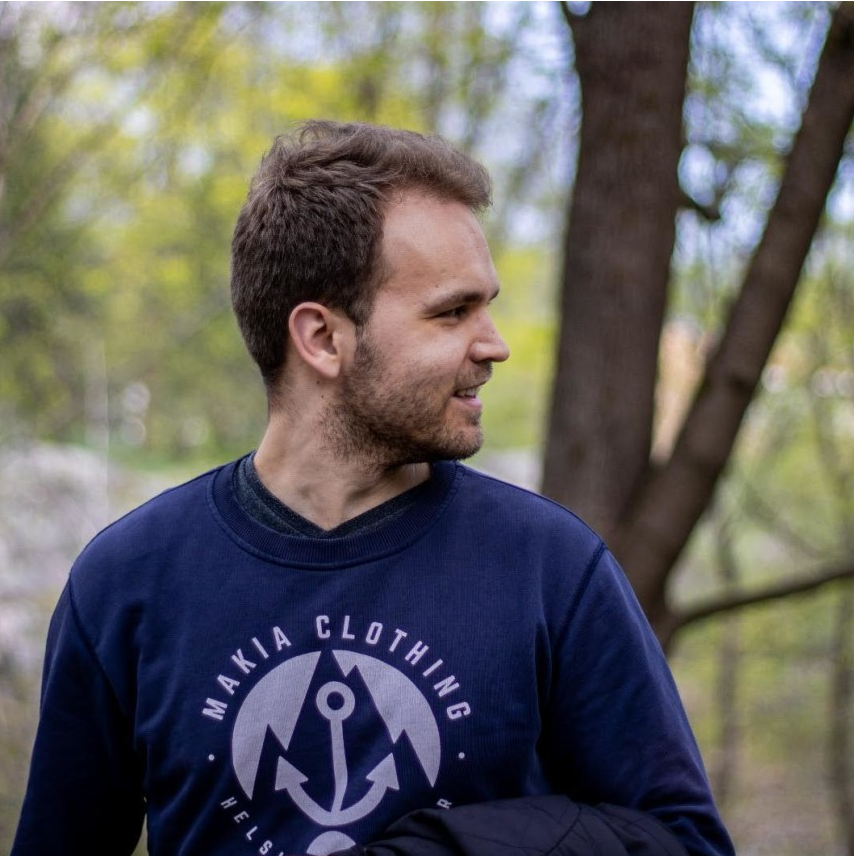
Veikko Karsikko,
Developer, AI Evangelist